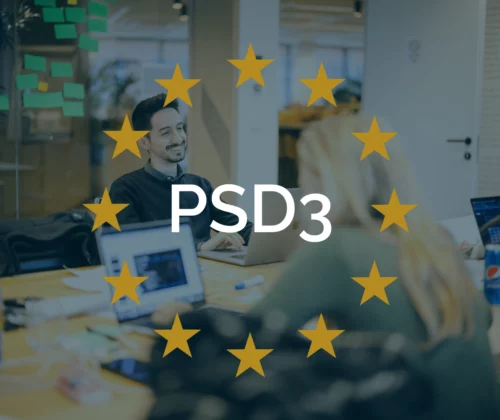
No financial institution escapes the supervision of the Money Laundering and Terrorist Financing Act (Wwft). After ING and ABN Amro were fined by De Nederlandsche Bank (DNB) in connection with shortcomings under the Wwft, Rabobank has now also received a proposed designation. To meet the obligations arising from the Wwft, among other things, banks have invested large amounts of money and manpower in improving their transaction monitoring. Because of the large number of transactions, efficiency remains important. Therefore, traditional methods such as simple business rules are increasingly being traded in for complex, sophisticated machine learning techniques.
In the previous article on payment institutions and the Wwft, we touched on a number of challenges that payment institutions may encounter when dealing with the Wwft and the Sanctions Act 1977 (SW). Among other things, the creation of a transaction profile and its monitoring were discussed.
In this second article, Mark Croes of RiskQuest and Remco Voogt of Projective Group take a closer look at transaction monitoring and recent developments within this field. We will see that there are still big steps to be made for payment institutions. This is also an opportunity to make use of the 'lessons learned' at banks, and to set up transaction monitoring properly and efficiently right away. In this way, payment institutions can remain efficient and maintain competitive advantages.
Transaction monitoring can be divided into two categories:
The first category primarily uses systems that compare transactions to sanction lists. For this purpose, origin, destination, description, among others, are analyzed and compared with sanction lists. The challenge here lies, for example, in speed - after all, these are transactions that need to be executed quickly and thus checked quickly. Another challenge is analyzing the text. After all, if a transfer with description "water tanks" is made it may be legitimate, while a description "tanks" may have to be blocked. In addition, there are different ways of spelling and misspelling that add to the complexity.
In the remainder of this article, we will focus mainly on the second category, post-event transaction monitoring. This refers to everything that is done after the transaction has already taken place. Once client transactions have been processed, various methods can be used to check for possible "unusual transaction patterns and client transactions, which by their nature pose a higher risk of money laundering or terrorist financing." [2]
The post-transaction monitoring landscape has seen a period of innovation in recent years due to increased investment. Previously, business rules (decision rules) were primarily used to flag potentially suspicious behavior. Here, for example, a signal is given when a customer spends more than X amount of cash.
The disadvantage of these business rules is that only known forms of money laundering, terrorist financing, etc. are detected. After all, a specific business rule must be written that then marks only those transactions as potentially suspicious. In addition, it is a lot of work to keep track of all these business rules, as they are essentially large fishing nets to catch certain behaviors. However, experience shows that it takes a lot of manpower to pick out the real cases of money laundering or terrorist financing. For some time now, therefore, machine learning techniques have been used in which a complex algorithm ensures that a computer can recognize certain behavior. Within machine learning, we distinguish between two major streams: supervised and unsupervised.
The disadvantage of business rules is that only known forms of money laundering and terrorist financing are detected.
Supervised learning uses data with a label. The label indicates whether certain behavior is undesirable or not. These labels are available if there is historical data that has been examined for money laundering, terrorist financing or other undesirable behavior. The computer then learns to make a connection between the data and the label. This data can consist of all kinds of "features" that describe transaction behavior. For example, the number of international transactions a customer makes in a period of time, but also features that describe the customer himself, such as age. The algorithm then learns to make a connection between the labels and these features. The trained model can then be applied to new transactions to recognize undesirable behavior.
Now, a payment institution may not have historical data with known instances of lawbreaking behavior. Or it may be looking for as yet unknown forms of such behavior. This is where unsupervised learning can be used. Unsupervised learning involves algorithms that learn without using labels. Here it is not known which historical transactions are undesirable, but the algorithm learns what forms of "normal" behavior are. Indeed, the bulk of customers do not engage in criminal transactions; the algorithm will learn that this is normal behavior. Any deviations from this will be flagged by the algorithm as unusual. This does not mean that it is automatically money laundering or other criminal behavior, but it does mean that it is worth investigating further
As the number of fintechs such as payment service providers increases, so will the amount of data available to these service providers. At the same time, the need for a proper transaction monitoring framework at these service providers will also increase. After all, they are now an important link in the transaction landscape and must ensure that their services are not used by criminals. This provides both opportunities and urgency to take big steps in establishing this framework. Already in 2016, the DNB conducted a thematic study "post-event transaction monitoring at payment institutions," and the focus on these payment institutions will not diminish in the near future. The developments at the aforementioned banks show this convincingly.
Payment institutions still have big steps to take in the field of transaction monitoring. This is precisely why it is important to make use of 'lessons learned' from banks.
Payment institutions therefore still have big steps to take in the field of transaction monitoring. This is precisely why it is important to make use of 'lessons learned' from banks. This way, payment institutions do not have to step into the same pitfalls. Here are a few examples of these lessons learned
An important lesson is to use machine learning techniques to reduce the time-consuming work of creating and maintaining business rules. Here, it is not recommended to replace business rules, but a combination of both techniques is ideal in this regard. As discussed, in the early stages unsupervised will be more obvious given the amount of labeled data.
It is also possible for payment institutions to use intelligent models to recognize certain suspicious patterns among customers. This could include merchants or Web shops where customers often use multiple payment service providers within a single transaction. This adds an extra layer so that the origin of the transaction is even more difficult to trace. If this happens remarkably often at a particular merchant, it may indicate that this merchant is not acting bona fide. In this way, transaction monitoring can strengthen customer research.
As data on criminal behavior and parties involved expands, it can be used for network analysis. This allows payment institutions to identify possible clusters involved in criminal behavior. In an ideal situation, these insights are also shared among payment institutions themselves or with traditional banks. This makes it easier to detect rogue entities, and at potentially lower cost.
In addition to learning from the best practices of traditional banks, payment institutions can also take advantage of the benefits PSD2 brings. The introduction of PSD2 allows payment institutions to do analysis on historical banking transactions with the consent of this potential customer. This will allow them to screen prospective customers already during the onboarding process and establish an expected transaction profile. This transaction profile will then serve to better recognize deviant behavior from the expected profile. An intelligent tool that can be used for this purpose is the RiskQuest Navigator, which provides insight into the customer's profile during the screening process.
Fintechs, including payment institutions, can thus learn a lot from the developments in transaction monitoring at banks. It is important to get transaction monitoring right from the start to create an efficient and scalable system that allows fintechs to maintain their competitive advantages.
At the same time, it is important to pay attention to the specific risks and characteristics that payment institutions face, such as the international nature of transactions. Payment institutions will in certain cases have to make different choices in this than banks, for example because in certain cases the payment institution has more or less information available.
So there are various challenges for payment institutions in the area of transaction monitoring. To remain efficient, it is very important that the transaction monitoring framework is set up properly from the very beginning. RiskQuest has extensive experience in setting this up. Their tool, the RiskQuest Navigator, helps screen clients based on transaction data.
Projective Group can help you adequately implement the Wwft requirements from a legal perspective and translate this to your daily practice. We also offer a Transaction Monitoring e-learning. This training increases your understanding of the legal requirements in the field of transaction monitoring under the Wwft, and helps you practice the skills you need to effectively monitor transactions.
Would you like to know more about this?